Data Landscape and Trending in Data Solution in 2023
This article will bring you three notable data solution trends in 2023 related to Unified Data Platform, DataOps and Data Mesh.
Bài viết này có phiên bản Tiếng Việt
When I began working with the team to build a skill map for data platform in 2020, I was overwhelmed by the diversity and variaty of choices, from building to buying components for the data solution architecture of our enterprise. Today's technology enables the handling of large data problems that were still research topics 15-20 years ago. However, the influx of money into technology startups also fragments this landscape and creates a FOMO syndrome within the data industry.
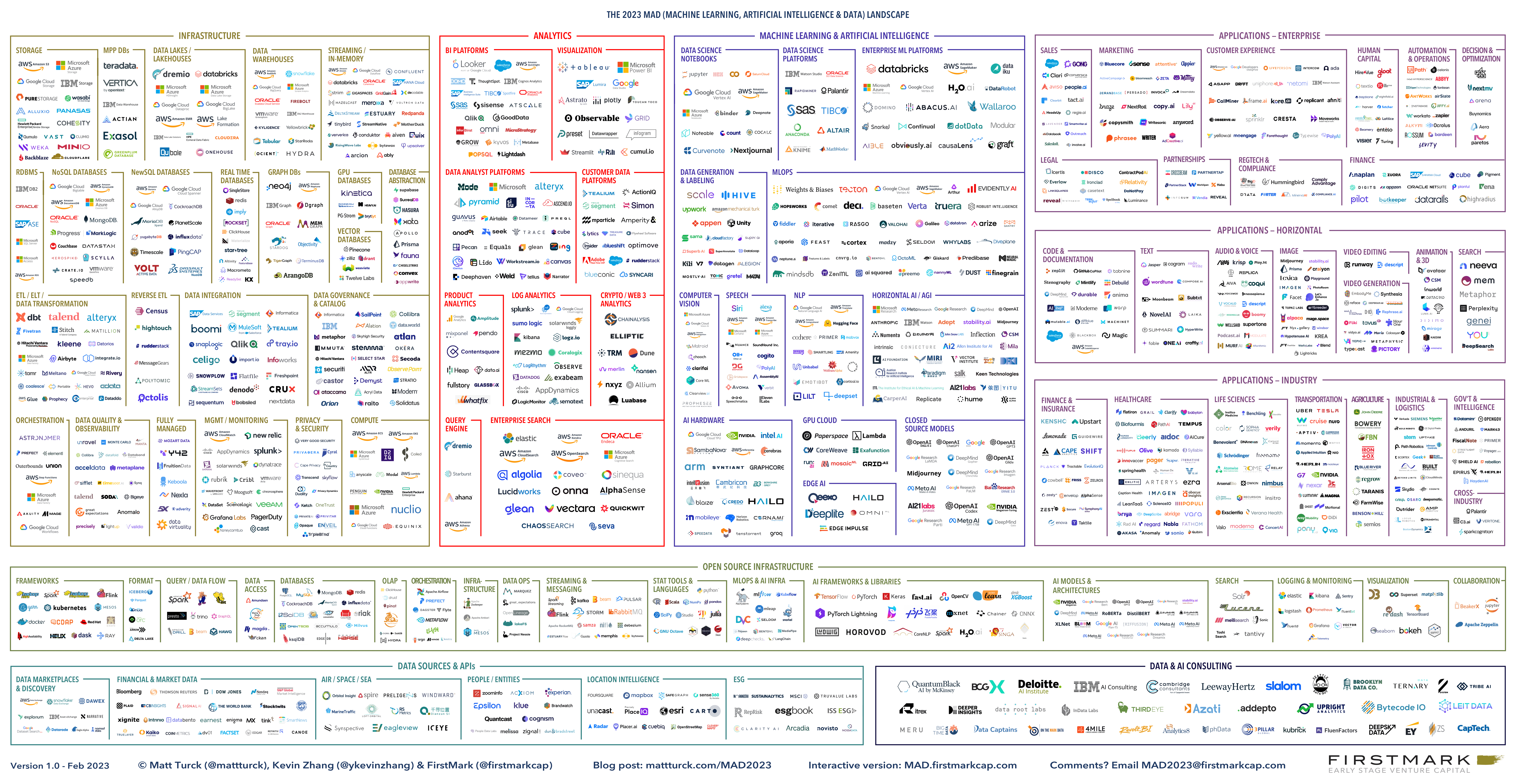
Although we hope that the future will make these technologies truly easy to integrate or unify into standardized platforms, for now, we still have to accept the diversity and make appropriate choices in building data platforms and the data capabilities of our engineering team. From open source to expensive platforms, from neat products, each solution has its own strengths and still has its loyal engineers. The important criterion for building a data system is still closely linked to the business results of the enterprise, while technology will be the second factor to consider.
It is not uncommon in the technology industry to have a new buzzword to sell every year. If data streaming was the hot keyword before, now it is DataOps, Data Mesh, and even Data Fabric. To choose the appropriate technology for the problem, there needs to be an objective approach with full information evaluation according to a framework that includes:
- Strategy: Business strategy and data strategy
- Technology: Technology efficiency, integration and popularity
- People: Happiness that data brings
Depending on which components you want to start building from, the article suggests some notable trends that you can start with from the above picture:
Unified Data Platform - Ambitious to create a unified platform
A unified data platform has many benefits. One of them is reducing development costs for data collection. It also helps accelerate application development on the platform and has good scalability. In addition, a unified system helps reduce capital costs (Capex), is flexible in upgrading and downgrading, helps analyze data better, and supports better decision-making to drive business growth and profitability.
Therefore, the deployment of complex systems with many tools has gradually been replaced by the trend of creating a unified platform that provides many features. The ability to integrate with various different tools is also a necessary requirement for implementation into existing systems.
Unified data platforms allow indexing and integration of important data, preparing and cleaning data quickly at an unlimited scale. In addition, it allows for continuous training and deployment of machine learning models for artificial intelligence applications.
Some notable platforms are Databricks' Unified Data Analytics Platform, which helps organizations accelerate innovation by merging data science with technology and business, or Google Cloud’s Unified Analytics Data Platform, which allows users to access data in data warehouses without affecting the performance of other tasks accessing that data warehouse. A leading tool in this trend is Azure Synapse Analytics, introduced with customization and flexible deployment models along with an interactive interface that has ambitious goals for a unified solution for processing big data.
DataOps - Designing processes and automation for data analytics
During the development and expansion of a business, the data system will face certain challenges: using multiple tools in data processing leading to technology overload; Data teams have experts from different fields, each role has different requirements and needs for the system and data; The explosion in size and complexity of data over time. These challenges are the premise for DataOps.
DataOps refers to methods that bring certain benefits to the data system such as speed, flexibility, quality assurance, compliance with data protection regulations... to help stakeholders, such as IT system operators, data analysts, data engineers... can communicate easily with each other.
Some examples of DataOps are: Automated data validation upon entering the system and after each transformation step, notifying the data team in case of errors, automating system operation and being able to customize for each case, all system changes can be version controlled...
Some tools that support the DataOps trend can be mentioned such as Great Expectations - a tool that supports setting "expectations" to check the quality of data, lakeFS - a version control tool for data lakes, allowing the ability to "re-run" old experiments during development.
Modern "toolkits" also allow for DataOps implementation, such as Azure Data Factory simplifying pipeline building with a visual interface, integrating with GitHub / Azure DevOps to store artifacts and version control,...
Data Mesh - A distributed data processing architecture model
To extract value, raw data always needs to go through stages such as sorting, filtering, preprocessing, etc. When scaling up the system, data is extracted from more sources and requires specialized transformations in different ways. This requires a team with knowledge in many data domains, able to quickly respond to changes and customizations in the processing system, as well as to evaluate the value of data to provide.
To meet this need, the Data Mesh model was born. In Data Mesh, domain ownership - people who own and understand their data very well - will be responsible for analyzing, processing and providing data to the system. Building, executing, maintaining, and exploiting data will be done through features and tools on a platform. Standardizing data to comply with rules and regulations will be done by an administration team.
Applying Data Mesh in a business brings benefits to the data system such as increasing the value of data exploitation, increasing flexibility, increasing efficiency on costs, enhancing security, and compliance.
Tools to serve the construction of Data Mesh: Azure Synapse Analytics, Google Cloud BigQuery, AWS Lake Formation - AWS Data Exchanges - AWS Glue, dbt and Snowflake, Databricks...
Source: Mattturck